Creating a Responsible AI Policy for Public Entities
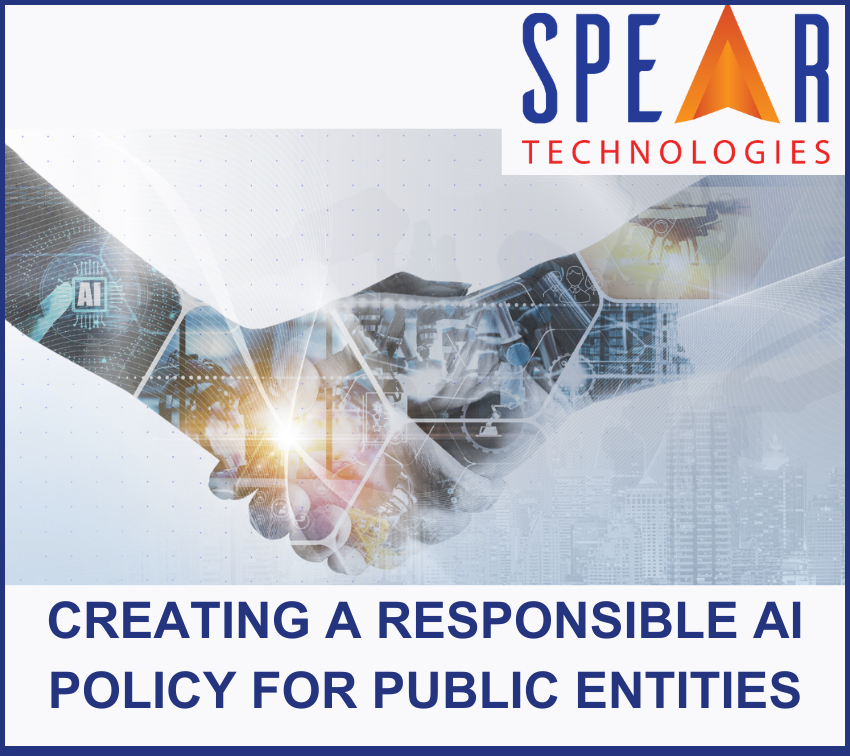
Public entities are adopting AI technologies at a record pace. Recent statistics show that 42% of public entities have deployed AI in some form, with another 40% currently exploring the technology1. The benefits of adopting AI are well documented: improved efficiency, reduced costs, and enhanced service delivery.
Despite the growth and uptake, there are challenges that need to be navigated along with adoption. Factors to consider include data privacy concerns, security concerns, ethical and bias concerns, trust and transparency issues, as well as potential data complexities. Also consider that Generative AI models with advanced capabilities such as content and code generation, summarization, and search also come with increased responsible AI challenges related to harmful content, manipulation, human-like behavior, privacy, and more.
A well-crafted organizational AI policy can address these challenges and mitigate concerns across the organization. Creating effective artificial intelligence (AI) policies for public entities involves addressing ethical, legal, and operational considerations to ensure AI technologies are used responsibly, transparently, and for the public good. Below are key components and guidelines for developing such policies:
- Ethical Guidelines
- Fairness and Non-Discrimination: Ensure AI systems do not perpetuate or exacerbate biases. Implement measures to identify and mitigate bias in data and algorithms.
- Transparency and Explainability: AI systems should be transparent in their decision-making processes. Public entities should be able to explain how decisions are made and provide documentation on the AI models used.
- Accountability: Define clear lines of accountability for AI decisions. Establish mechanisms for redress in case of harm caused by AI systems.
- Privacy and Data Protection: Ensure that AI systems comply with data protection laws and prioritize the privacy of individuals. Implement data minimization and secure data handling practices.
- Legal and Regulatory Compliance
- Adherence to Laws and Regulations: Ensure AI deployment complies with existing laws, including those related to data protection, discrimination, and safety.
- Intellectual Property: Address issues related to the ownership and use of AI-generated outputs and the intellectual property of AI technologies.
- Intellectual Property: Address issues related to the ownership and use of AI-generated outputs and the intellectual property of AI technologies.
- Liability Framework: Establish a clear framework for liability in cases where AI systems cause harm or errors.
- Operational Policies Compliance
- Governance Structure: Create a governance framework for AI initiatives, including roles and responsibilities for oversight, management, and review.
- Risk Management: Implement risk assessment and management procedures to identify, evaluate, and mitigate potential risks associated with AI deployment.
- Continuous Monitoring and Evaluation: Regularly monitor AI systems for performance, fairness, and compliance with ethical standards. Implement mechanisms for continuous improvement based on feedback and new developments.
- Public Engagement and Education
- Stakeholder Involvement: Engage with a broad range of stakeholders, including the public, to gather input and build trust in AI initiatives.
- Public Education: Provide resources and programs to educate the public about AI, its benefits, and potential risks.
- Research and Development
- Encourage Innovation: Promote research and development in AI to foster innovation while ensuring alignment with ethical and legal standards.
- Collaboration: Foster collaboration between public entities, academia, industry, and other stakeholders to advance AI research and best practices.
- Procurement and Vendor Management
- Responsible Procurement: Ensure that AI systems procured from third parties meet ethical, legal, and technical standards. Include clauses in contracts to enforce compliance.
- Vendor Transparency: Require vendors to provide detailed information about their AI systems, including data sources, algorithms, and potential biases.
- Interagency and International Cooperation
- Interagency Collaboration: Facilitate cooperation between different public entities to share knowledge, resources, and best practices in AI deployment.
- International Standards: Align with international standards and guidelines on AI to ensure global consistency and cooperation.
Once the framework of your AI policy is developed, implementation is the next crucial step. Here are the steps to effectively implement your AI policy:
Implementation Steps for AI Policy
- Communicate the Policy
- Internal Communication: Clearly communicate the policy to all stakeholders within the organization. Ensure that everyone, from top management to front-line employees, understands the policy’s objectives, guidelines, and their roles in its implementation.
- External Communication: If applicable, communicate the policy to external stakeholders, including vendors, partners, and the public. This helps build trust and transparency.
- Train Employees
- Training Programs: Develop and conduct comprehensive training programs to educate employees about AI technologies, ethical considerations, legal compliance, and their specific responsibilities under the new policy.
- Ongoing Education: Implement continuous learning opportunities to keep staff updated on the latest developments in AI and relevant regulations.
- Establish Governance and Oversight
- Governance Structures: Set up governance structures as outlined in your policy. This includes appointing AI ethics boards, compliance officers, and creating committees for oversight and review.
- Accountability Mechanisms: Define clear lines of accountability. Ensure there are designated individuals responsible for monitoring compliance, assessing risks, and addressing any issues that arise.
- Develop Standard Operating Procedures (SOPs)
- Detailed SOPs: Create detailed SOPs for the deployment and use of AI technologies. These should cover all aspects of AI operations, from data collection and processing to decision-making and user interactions.
- Integration with Existing Processes: Integrate these SOPs with existing organizational processes to ensure seamless implementation.
- Monitor and Evaluate
- Continuous Monitoring: Implement systems for continuous monitoring of AI applications. This includes tracking performance, identifying potential biases, and ensuring compliance with ethical and legal standards.
- Feedback Mechanisms: Establish mechanisms to collect feedback from users and stakeholders. Use this feedback to make necessary adjustments and improvements.
- Risk Management
- Risk Assessment: Conduct regular risk assessments to identify and mitigate potential risks associated with AI deployment. This includes evaluating the impact on privacy, security, and ethical considerations.
- Incident Response: Develop and implement an incident response plan to address any issues or breaches that occur. Ensure that there are clear protocols for reporting and resolving incidents.
- Engage with Stakeholders
- Stakeholder Engagement: Maintain ongoing engagement with stakeholders to build trust and ensure that their concerns are addressed. This includes regular meetings, public consultations, and transparency reports.
- Public Awareness: Run public awareness campaigns to educate the community about the benefits and risks of AI, and how the organization is addressing them.
- Evaluate and Update Policy
- Periodic Review: Regularly review and update the AI policy to reflect new developments in technology, regulations, and organizational goals. Ensure that the policy evolves with changing circumstances.
- Benchmarking: Compare your policy and its implementation against industry standards and best practices. Use benchmarking to identify areas for improvement.
By following these steps, public entities can ensure that their AI policies are not only well-crafted but also effectively implemented, leading to responsible and beneficial use of AI technologies.
It is imperative to get it right the first time, given the complexity and potential impact of AI implementation. As a leading provider of core P&C insurance software solutions with built-in AI, Spear recommends adhering to the best practices outlined in the Responsible AI Standard2. This standard breaks down the process into manageable steps, asking teams to Identify, Measure, Mitigate potential harms, and plan for how to Operate the AI system. In alignment with these practices, here are four key stages to consider:
- Identify: Prioritize potential harms from your AI system through iterative red-teaming, stress-testing, and analysis.
- Measure: Establish clear metrics to measure the frequency and severity of those harms, and complete systematic testing (both manual and automated).
- Mitigate: Implement tools and strategies to mitigate harms, such as prompt engineering and using content filters. Repeat measurements to test effectiveness post-mitigation.
- Operate: Define and execute a deployment and operational readiness plan.
Spear’s solutions with built-in AI offer an extra measure of control by limiting usage to client-specific data, preventing it from being shared into the AI tool’s master dataset, which is crucial for setting up a responsible AI policy.
To see first-hand how your organization can benefit from the adoption of AI that fosters the creation of a responsible AI policy with SpearClaimsTM, our award-winning claims system built by industry experts on a modern low code platform that delivers the power of built-in AI and Analytics while lowering your total cost of ownership, Schedule a Demo.
To discover how Spear’s solutions are accessible to insurers of all sizes, Request Pricing.
1According to findings from the IBM Global AI Adoption Index 2023
2Microsoft Responsible AI Transparency Report