6 Ways Machine Learning is Transforming Insurance
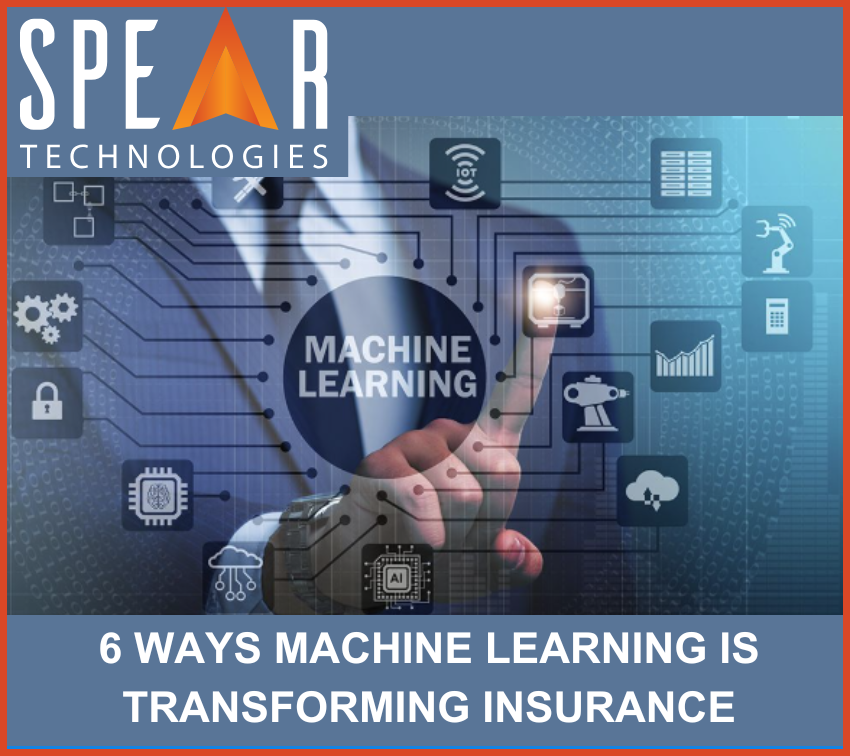
Machine Learning in Insurance
The insurance industry is undergoing rapid transformation, and Machine Learning (ML) is transforming insurance at the forefront of this evolution. By leveraging advanced algorithms and vast amounts of data, ML enables insurers to automate processes, improve risk assessment, and enhance customer engagement. Unlike traditional statistical models, ML continuously learns and adapts, making it a powerful tool for improving decision-making and operational efficiency.
When implemented effectively, ML helps insurers reduce costs, boost profitability, and deliver superior service to policyholders.
Key Applications of Machine Learning in Insurance
- Automated Risk Assessment: Enhances underwriting accuracy with real-time data insights.
- Fraud Detection: Identifies suspicious patterns in claims and applications.
- Claims Processing Optimization: Speeds up settlements through intelligent automation.
- Customer Personalization: Offers tailored policies and services based on behavioral data.
- Dynamic Pricing Models: Adjusts premiums based on real-time risk factors.
By embedding ML into their core processes, insurers can drive innovation and efficiency across the value chain.
How Machine Learning is Transforming Insurance
Here’s how insurers can leverage ML to improve customer experience and operational performance:
1. Enhancing Risk Assessment and Underwriting
Underwriting relies on data-driven insights, and ML enhances this process by:
- Analyzing vast datasets, including IoT, telematics, and third-party data sources.
- Predicting potential claims risks more accurately.
- Refining pricing models based on granular risk factors.
For example, auto insurers can use ML to analyze real-time driving behavior from connected vehicles, enabling personalized premium adjustments based on individual risk profiles.
2. Detecting and Preventing Fraud
Insurance fraud costs the industry billions annually, but ML enhances fraud detection by:
- Identifying anomalies and suspicious claims patterns.
- Using predictive models to flag high-risk transactions.
- Enhancing fraud detection capabilities with continuous learning algorithms.
By integrating ML-powered fraud detection, insurers can proactively reduce fraudulent claims and associated financial losses.
3. Automating Claims Processing and Settlement
Claims processing is one of the most critical functions in insurance. ML optimizes this process by:
- Automating claims triage and prioritization.
- Predicting claim complexity and recommending the appropriate handling approach.
- Reducing manual processing time, improving efficiency and accuracy.
For instance, ML can determine whether a claim requires manual review or can be settled instantly, leading to faster resolutions and improved policyholder satisfaction.
4. Improving Customer Retention and Personalization
Retaining policyholders is crucial, and ML enables insurers to:
- Identify customers likely to churn and offer proactive retention strategies.
- Provide personalized policy recommendations based on customer data.
For example, P&C insurers can use ML to assess property risk factors, such as weather patterns or maintenance history, and recommend appropriate coverage adjustments.
5. Implementing Usage-Based and Behavioral Pricing
Traditional insurance pricing models often rely on broad risk categories, but ML allows insurers to:
- Develop dynamic pricing models that adjust in real time.
- Offer usage-based insurance (UBI) by analyzing telematics and behavioral data.
- Provide incentives for risk-mitigating behaviors, such as safe driving habits.
For example, insurers can use ML-driven insights from smart home devices to offer discounts to homeowners who implement safety measures, such as water leak detectors or security systems.
6. Strengthening Regulatory Compliance and Risk Management
Compliance remains a top priority for insurers, and ML aids in regulatory adherence by:
- Automating compliance monitoring and reporting.
- Identifying potential regulatory risks before they escalate.
- Enhancing transparency and auditability with AI-driven documentation.
By integrating ML into compliance workflows, insurers can reduce legal risks while maintaining industry standards and consumer trust.
Real-World Applications in Insurance
Leading insurers are already leveraging ML to enhance their operations:
- AI-Driven Risk Scoring: Improves underwriting efficiency and risk accuracy.
- Automated Claims Adjudication: Accelerates settlements while reducing manual effort.
- Customer Lifetime Value Prediction: Helps insurers tailor services to high-value policyholders.
Challenges and Considerations
While ML presents immense opportunities, insurers must address key challenges:
- Data Quality and Integration: Ensuring clean, structured, and well-integrated data.
- Privacy and Security: Safeguarding customer data while adhering to regulations.
- Algorithm Bias and Explainability: Mitigating biases in AI-driven decisions and ensuring transparency.
Conclusion
Machine Learning is revolutionizing the insurance industry by enabling smarter decision-making, enhancing customer experiences, and optimizing operations. By strategically implementing ML-driven solutions, insurers can gain a competitive advantage, improve profitability, and better serve their policyholders. As technology advances, ML will continue to shape the future of insurance, making now the ideal time to embrace its potential.
Schedule a Demo to explore how SpearSuite™, our award-winning P&C insurance software suite, integrates AI-driven machine learning capabilities for smarter underwriting, fraud detection, and claims automation.
To discover how Spear’s solutions can help insurers of all sizes, Request Pricing today.